Learning to Control in Power Systems: Design and Analysis Guidelines for Concrete Safety Problems
Patricia Hidalgo-Gonzalez – University of California, San Diego, United States
Rapid progress in machine learning and artificial intelligence (AI) has brought renewed attention to its applicability in power systems for modern forms of control that help integrate higher levels of renewable generation and address increasing levels of uncertainty and variability. In this paper we discuss these new applications and shine light on the most relevant new safety risks and considerations that emerge when relying on learning for control purposes in electric grid operations. We build on recent taxonomical work in AI safety and focus on four concrete safety problems. We draw on two case studies, one in frequency regulation and one in distribution system control, to exemplify these problems and show mitigating measures. We then provide general guidelines and literature to help people working on integrating learning capabilities for control purposes to make safety risks a central tenet of design.
Bio: Dr. Patricia Hidalgo-Gonzalez is an Assistant Professor at the University of California, San Diego. She is an NSF GRFP fellow, Siebel Scholar in Energy, Rising Star in Electrical Engineering and Computer Science, and has been awarded Best paper at the Power Systems Computation Conference 2020, the UC Berkeley Graduate Opportunity Program Award, and the Outstanding Graduate Student Instructor Award. At UC San Diego, she directs the Renewable Energy and Advanced Mathematics (REAM) lab which focuses on high penetration of renewable energy using optimization, control theory and machine learning. Dr. Hidalgo-Gonzalez co-developed a stochastic power system expansion model to study the Western North America’s grid under climate change uncertainty. She is currently working with the California Energy Commission to understand the value and role of long-duration energy storage. She is also supported by the U.S. Department of Energy to study the value of wave energy and offshore wind for the U.S. power grid. In addition to capacity expansion modeling, she works on power dynamics with low and variable inertia, and controller design using machine learning and safety guarantees. She is generally interested in power dynamics, electricity market redesign to aid the integration of renewable energy, microgrids for wildfire risk mitigation, distributed control, and learning for dynamical systems with safety guarantees. Dr. Hidalgo-Gonzalez is part of the IEEE Power & Energy Society Task Force titled “Data-Driven Controls for Distributed Systems.” She holds two M.S. and a Ph.D. from UC Berkeley.
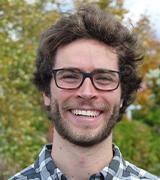
Location
Montréal Québec
Canada