Stochastic Control with Partial Information: Regularity, Optimality, Approximations, and Learning
Serdar Yüksel – Queen's University, Canada
Partially observed stochastic control provides a general model for many applications. In this seminar, we will first present a general introduction and then study regularity, optimality, approximation, and learning theoretic results for such problems.
The study of partially observed models has in general been established via reducing the original partially observed stochastic control problem to a fully observed one with probability measure valued filter (or belief) states and an associated filtering equation forming a Markovian kernel. We will first establish regularity results for this kernel, involving weak continuity as well as Wasserstein regularity and contraction, and present existence results for optimal solutions for both the discounted cost (under weak continuity) and average cost (under Wasserstein regularity and contraction) criteria.
Building on these, we then present approximation results via either quantized (probability-measure valued) filter approximations or finite sliding window approximations under filter stability: Filter stability refers to the correction of an incorrectly initialized filter for a partially observed dynamical system with increasing measurements. We present explicit conditions for controlled filter stability which are then utilized to arrive at near-optimal finite-window control policies by viewing truncated memory as a uniform quantization of an alternative filter state reduction consisting of the prior at a past time stage and the following finite memory.
Finally, we establish the convergence of a reinforcement learning algorithm for control policies using these finite approximations or finite window of past observations (by viewing the quantized filter values or finite window of measurements as ‘states’) and show near optimality of this approach under explicit conditions. While there exist many experimental results, (i) the rigorous asymptotic convergence for such finite-memory Q-learning algorithms, and (ii) the near optimality with an explicit rate of convergence (in the memory size) are new to the literature. As a corollary, this analysis establishes near optimality of classical Q-learning for continuous state space stochastic control problems (by lifting them to partially observed models with approximating quantizers viewed as measurement kernels) under weak continuity conditions. Extensions of the above for average cost criteria (for learning and robustness), and a general class of non-Markovian systems will also be presented. (Joint work with Ali D. Kara).
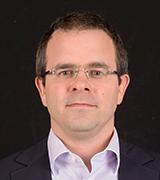
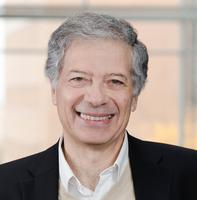
Lieu
Pavillon André-Aisenstadt
Campus de l'Université de Montréal
2920, chemin de la Tour
Montréal Québec H3T 1J4
Canada