OR@Africa Annual Day
Link to participate with Zoom
To mark OR@AFRICA Day, we're delighted to be hosting a hybrid event at GERAD, bringing together professors, researchers and students with links to Africa to share their experiences, ideas and perspectives on Operations Research (OR). Guest speakers, including professors and PhD students, will present their work with a focus on bridging the gap between theory and practical applications in industries across Africa. The event aims to foster networking around African OR topics, provide participants with valuable feedback on emerging challenges, showcase innovative methodologies and explore future directions for OR to support sustainable development across the continent.
Registration is required to attend the event.
8h30-9h00 -- Reception
9h00-10h00 -- Session 1 (Introduction)
- Guest of honor: Professor Gilbert Laporte
- Presentation of OR@ Africa
- Acknowledgements
10h00-10h15 -- Break
10h15-12h00 -- Session 2 (Professors presenting articles)
- Professor François Soumis
- Professor Hanane Dagdougui
- Professor Nadia Lahrichi, Polytechnique Montréal
Application of OR tools to flow optimization in radiotherapy
The main cancer treatments are surgery, radiation therapy and chemotherapy. The complexity of the logistical process of scheduling treatment appointments stems from the fact that it involves extremely costly resources, sometimes synchronously. Several due dates (i.e., appointments already scheduled, maximum wait times) and unexpected events such as the arrival of patients requiring urgent palliative care add to the difficulty. This talk will investigate how can simulation and optimization models help improve the efficiency of cancer treatment centers and share experiences on patient booking, physician scheduling, and capacity assessment.
12h00-13h30 -- Lunch
13h30-15h15 -- Session 3 (Papers presented by OR researchers)
Ibrahim Dan Dije, UQAM
A 3-space dynamic programming heuristic for the cubic knapsack problem
The cubic knapsack problem (CKP) is a combinatorial optimization problem, which can be seen both as a generalization of the quadratic knapsack problem (QKP) and of the linear Knapsack Problem (KP). This problem consists of maximizing a cubic function of binary decision variables subject to one linear knapsack constraint. It has many applications in biology, project selection, capital budgeting problem, and in logistics. The QKP is known to be strongly NP-hard, which implies that the CKP is also NP-hard in the strong sense. Unlike its linear and quadratic counterparts, the CKP has not received much of attention in the literature. Thus the few exact solution methods known for this problem can only handle problems with up to 60 decision variables. In this paper, we propose a deterministic dynamic programming-based heuristic algorithm for finding a good quality solution for the CKP. The novelty of this algorithm is that it operates in three different space variables and can produce up to three different solutions with different levels of computational efforts. The computational results show that our algorithm can find optimal solutions for nearly 98\% of the test instances that could be solved to optimality. It also has the merit of finding, in less than five minutes, feasible solutions that cannot be outperformed by commercial solvers in 5 hours. The algorithm also empirically dominates the other existing heuristic algorithm for CKP.Awa khouna, Polytechnique Montréal
Stealing Trees Ensembles exploiting optimal counterfactual explanations
The rise of Machine Learning as a Service (MLaaS) has made advanced machine learning tools widely accessible, challenging the balance between model explainability and security. As MLaaS simplifies the use of complex models, the need for explainability—to make model decisions transparent and trustworthy—intensifies the risk of model extraction attacks. This work delves into the pressing issue of such attacks within the realm of machine learning, specifically targeting tree ensemble models using counterfactual explanations. Model extraction attacks pose a significant threat to the security and proprietary nature of machine learning models by enabling unauthorized parties to replicate a model with high fidelity without access to the original training data or the model architecture. Focusing on tree ensembles, a widely used model type for their simplicity and interpretability, we introduce a pioneering extraction attack algorithm: Tree Reconstruction Attack (TRA). This strategy exploits tree ensemble models' vulnerabilities by leveraging counterfactual explanations to deduce the model's structure and parameters. Notably, the TRA demonstrates a remarkable efficiency advantage, achieving model replication with 100% fidelity while requiring significantly fewer queries compared to existing benchmarks. This efficiency not only exposes a significant vulnerability but also emphasizes the potential for rapid dissemination of proprietary model details. Our approach demonstrates the ability to replicate any tree ensemble model accurately and efficiently, thereby underlining a critical security flaw in the deployment of these models and illustrating the trade-off between explainability and security.Sarah Nouri, Université des Sciences et de la Technologie Houari Boumediene
Two-Agent scheduling with time-dependent jobs and makespan as criterion
This study investigates the complexity of various time-dependent scheduling problems on a single machine involving two competing agents. Through the use of polynomial reductions and equivalences to established NP-Complete problems, we establish the NP-Completeness of several of these problems. Furthermore, we identify certain subproblems that can be solved in polynomial time and present methods for their resolution.
15h15-16h00 -- Cocktail
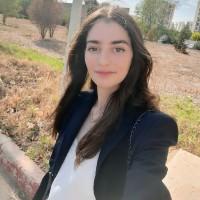
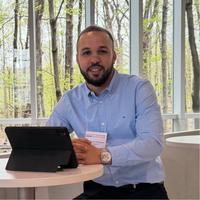
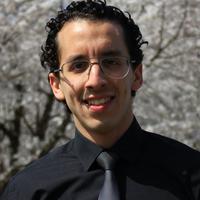
Location
André-Aisenstadt Building
Université de Montréal Campus
Montréal QC H3T 1J4
Canada