Planning and Learning in Risk-Aware Restless Multi-Arm Bandit
Nima Akbarzadeh – HEC Montréal, Canada
In restless multi-arm bandits, a central agent is tasked with optimally distributing limited resources across several bandits (arms), with each arm being a Markov decision process. In this work, we generalize the traditional restless multi-arm bandit problem with a risk-neutral objective by incorporating risk-awareness. We establish indexability conditions for the case of a risk-aware objective. In addition, we address the learning problem when the true transition probabilities are unknown by proposing a Thompson sampling approach and show that it achieves bounded regret that scales sublinearly with the number of episodes and quadratically with the number of arms. The efficacy of our method in reducing risk exposure in restless multi-arm bandits is illustrated through a set of numerical experiments.
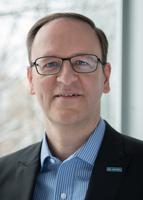
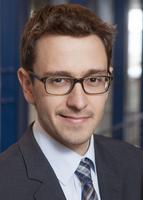
Lieu
Pavillon André-Aisenstadt
Campus de l'Université de Montréal
2920, chemin de la Tour
Montréal Québec H3T 1J4
Canada
Axe de recherche
Applications de recherche
- Énergie, environnement, ressources naturelles
- Infrastructures intelligentes (télécommunications, transport public, villes intelligentes)
- Ingénierie (conception en ingénierie, conception numérique)
- Logistique intelligente (conception d’horaires, chaînes d’approvisionnement, logistique, systèmes manufacturiers)
- Santé