Informed Posterior Sampling Based Reinforcement Learning Algorithms
Dengwang Tang – University of Southern California, États-Unis
Séminaire hybride à l'Université McGill ou Zoom.
In many traditional reinforcement learning (RL) settings, an agent learns to control the system without incorporating any prior knowledge. However, such a paradigm can be impractical since learning can be slow. In many engineering applications, offline datasets are often available. To leverage the information provided by the offline datasets with the power of online-finetuning, we proposed the informed posterior sampling based reinforcement learning (iPSRL) for both episodic and continuing MDP learning problems. In this algorithm, the learning agent forms an informed prior with the offline data along with the knowledge about the offline policy that generated the data. This informed prior is then used to initiate the posterior sampling procedure. Through a novel prior-dependent regret analysis of the posterior sampling procedure, we showed that when the offline data is informative enough, the iPSRL algorithm can significantly reduce the learning regret compared to the baselines (that do not use offline data in the same way). Based on iPSRL, we then proposed the more practical iRLSVI algorithm. Empirical results showed that iRLSVI can significantly reduce regret compared to baselines without regret.
Bio: Dengwang Tang is currently a postdoctoral researcher at University of Southern California. He obtained his B.S.E in Computer Engineering from University of Michigan, Ann Arbor in 2016. He earned his Ph.D. in Electrical and Computer Engineering (2021), M.S. in Mathematics (2021), and M.S. in Electrical and Computer Engineering (2018) all from University of Michigan, Ann Arbor. Prior to joining USC he was a postdoctoral researcher at University of California, Berkeley. His research interests involve control and learning algorithms in stochastic dynamic systems, multi-armed bandits, multi-agent systems, queuing theory, and game theory.
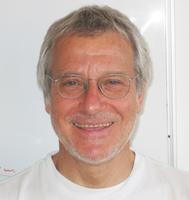
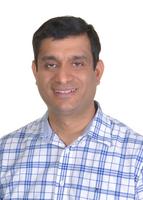
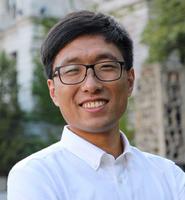
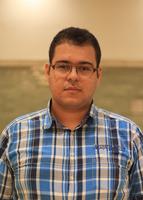
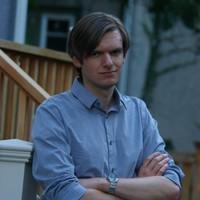
Lieu
CIM
Pavillon McConnell
Université McGill
Montréal QC H3A 0E9
Canada