Fast Continuous and Integer L-shaped Heuristics Through Supervised Learning
Emma Frejinger – Université de Montréal, Canada
Séminaire hybrique sur Zoom et dans la salle de séminaire du GERAD.
We propose a methodology at the nexus of operations research and machine learning (ML) leveraging generic approximators available from ML to accelerate the solution of mixed-integer linear two-stage stochastic programs. We aim at solving problems where the second stage is highly demanding. Our core idea is to gain large reductions in online solution time while incurring small reductions in first-stage solution accuracy by substituting the exact second-stage solutions with fast, yet accurate supervised ML predictions. This upfront investment in ML would be justified when similar problems are solved repeatedly over time, for example, in transport planning related to fleet management, routing and container yard management.
Our numerical results focus on the problem class seminally addressed with the integer and continuous L-shaped cuts. Our extensive empirical analysis is grounded in standardized families of problems derived from stochastic server location (SSLP) and stochastic multi knapsack (SMKP) problems available in the literature. The proposed method can solve the hardest instances of SSLP in less than 9% of the time it takes the state-of-the-art exact method, and in the case of SMKP the same figure is 20%. Average optimality gaps are in most cases less than 0.1%.
(Authors: Eric Larsen, Emma Frejinger, Bernard Gendron, Andrea Lodi)
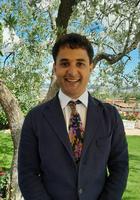
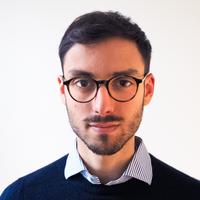
Lieu
Pavillon André-Aisenstadt
Campus de l'Université de Montréal
2920, chemin de la Tour
Montréal Québec H3T 1J4
Canada