A neural network approach for high-dimensional optimal control
Derek Onken – Emory University, États-Unis
Optimal control (OC) problems aim to find an optimal policy that control given dynamics over a period of time. For systems with high-dimensional state (for example, systems with many centrally controlled agents), OC problems can be difficult to solve globally. We propose a neural network approach for solving such problems. When trained offline in a semi-global manner, the model is robust to shocks or disturbances that may occur in real-time deployment (e.g., wind interference). Our unsupervised approach is grid-free and scales efficiently to dimensions where grids become impractical or infeasible. We demonstrate the effectiveness of our approach on several multi-agent collision-avoidance problems in up to 150 dimensions.
Bio: Derek Onken is a current PhD candidate in the Computer Science and Informatics Program at Emory University and is advised by Lars Ruthotto. Prior to joining Emory, he received his BS in Mathematics and Computer Science from the University of Georgia. His research examines design and applications of neural ordinary differential equations that arise from the blending of neural networks and differential equations. In addition to his dissertation research, Derek enjoys applying machine learning to applications arising in the health industry. He recently published work using cell phone billing records for privacy-preserving epidemic surveillance. In internships with the US Air Force Research Labs and UnitedHealth Group R&D, he applied machine learning methods to toxicology and lung cancer detection. He recently accepted a position at Eli Lilly and Co.
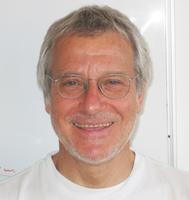
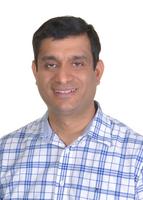
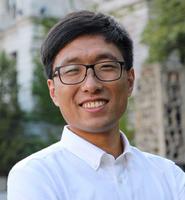
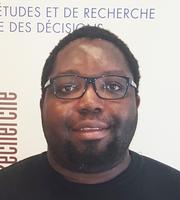
Lieu
Montréal Québec
Canada