Federated learning meets multi-objective optimization
Kiarash Shaloudegi – Huawei Noah’s Ark, Canada
Federated learning has emerged as a promising, massively distributed way to train a joint deep model over large amounts of edge devices while keeping private user data strictly on device. In this work, motivated from ensuring fairness among users and robustness against malicious adversaries, we formulate federated learning as multi-objective optimization and propose a new algorithm FedMGDA+ that is guaranteed to converge to Pareto stationary solutions. FedMGDA+ is simple to implement, has fewer hyperparameters to tune, and refrains from sacrificing the performance of any participating user. We establish the convergence properties of FedMGDA+ and point out its connections to existing approaches. Extensive experiments on a variety of datasets confirm that FedMGDA+ compares favorably against state-of-the-art.
Inscrivez-vous à la notification par e-mail des séminaires d'apprentissage automatique efficace du GERAD.
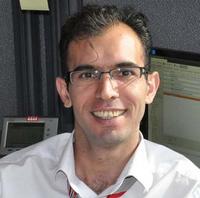
Lieu
Montréal Québec
Canada