Gaussian variational inference for batch nonlinear state estimation applied to robot navigation
James Richard Forbes – Professeur agrégé, Département de génie mécanique, Université McGill, Canada
For a robot to execute a meaningful task, three problems must be solved: navigation (where is the robot), guidance (where should the robot go), and control (what inputs should be applied). The navigation problem boils down to solving for the robot position and attitude, often considered the state of the robot, given noisy sensor data. The most popular solution to the navigation problem is the maximum a posterior probability (MAP) solution where the state that maximizes the posterior distribution of the states given the measurements is found. In practice, the MAP state estimate is found by solving a nonlinear least squares problem, and once the MAP state estimate has been found, the associated covariance is computed. From an optimization perspective, the design variable is the state estimate, and not the state estimate and the covariance. Motivated by a desire to find the Gaussian distribution that best approximates the posterior distribution, not just a best-fit state, this talk will explore how variational inference can be used for state estimation. In particular, starting with the Kullback-Leibler (KL) divergence between an assumed Gaussian distribution and the full posterior distribution of the state given the measurements, a recursive method to find the mean and covariance of the Gaussian distribution that minimizes the KL divergence will be presented. From an optimization point of view, the design variables are now both the mean and covariance associated with the state estimation problem, not just the mean, leading to an optimization problem with more design variables, and thus freedom to find a "best" navigation solution. This talk is based on the paper https://arxiv.org/pdf/1911.08333.pdf co-authored by Prof. T.D. Barfoot (U. Toronto), J.R. Forbes (McGill), and D.J. Yoon (U. Toronto).
Bienvenue à tous!
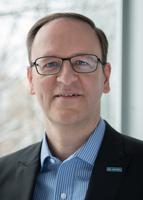
Lieu
Montréal Québec
Canada