Combinatorial optimization augmented machine learning for contextual multi-stage problems
Maximilian Schiffer – Professor of Operations and Supply Chain Management, TUM School of Management, Technical University of Munich, Germany
Combinatorial optimization augmented machine learning (COAML) is a novel field that combines methods from machine learning and operations research to tackle contextual data-driven problems that involve both uncertainty and combinatorics. These problems arise frequently in industrial processes, where firms seek to leverage large and noisy data sets to optimize their operations. COAML typically involves embedding combinatorial optimization layers into neural networks and training them with decision-aware learning techniques. This talk provides an overview of the underlying paradigm, algorithmic pipelines, and foundations based on selected application cases. Particularly, I will demonstrate the effectiveness of COAML on contextual and dynamic stochastic optimization problems, as evidenced by its winning performance on the 2022 EUROMeetsNeurIPS dynamic vehicle routing challenge.
Short Bio: Maximilian Schiffer is a tenured Professor (Universitätsprofessor W3) of Business Analytics & Intelligent Systems in the School of Management and a Core Member of the Munich Data Science Institute at the Technical University of Munich. Moreover, Maximilian is an Associate Member of the GERAD. Before joining TU Munich, he was a visiting postdoctoral scholar at Stanford University and a postdoctoral scholar at RWTH Aachen University. He received a Ph.D. degree in Operations Research from RWTH Aachen University in 2017. As a scholar, Maximilian’s expertise lies in the field of Computational Analytics, combining techniques from Operations Research, Prescriptive Analytics, Machine Learning, and Data Science. From a methodological angle, his research currently focuses on structured learning pipelines for online optimization, deep reinforcement learning, robust optimization, interpretable machine learning, as well as metaheuristics and exact combinatorial algorithms. Often, he applies these methodologies to develop decision support for the design and operations of (future) mobility and transportation systems or supply chains. He is the recipient of several awards, including the INFORMS TSL Dissertation Prize and the GOR Doctoral Dissertation Prize. His work has among others been recognized with a best paper award from the IEEEConference on Intelligent Transportation Systems, an Anna Valicek Medal from the AGIFORS, and a best paper award from the INFORMS TSL Society. He is currently serving on the Editorial Boards of Transportation Science, Service Science, OR Spectrum, Transportation Research Part C & Part E, and Business & Information Systems Engineering.
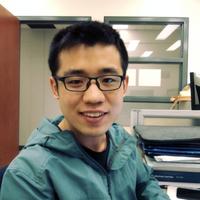
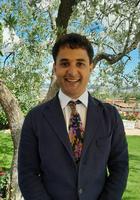
Location
Pavillon André-Aisenstadt
Campus de l'Université de Montréal
2920, chemin de la Tour
Montréal Québec H3T 1J4
Canada