A data-driven method for constraint customization in optimization models
Mahdis Bayani – Polytechnique Montréal, Canada
Decision-makers in different industries use optimization software for planning and decision-making. In numerous practical applications, these optimization tools are often not readily adjustable or configurable by end users due to limited knowledge, resources, or the required investment to consistently make such customizations. As a result, planners frequently adjust the solutions obtained from software based on implicit internal operational rules and preferences in order to make them feasible in practice. These practices may differ across various business units and subsidiaries. To ensure that such rules can be learned and systematically incorporated into optimization models, one can leverage data-driven methods to learn and embed implicit side constraints in a mixed integer linear program (MILP). These customization constraints can be created from machine learning models which are trained using previously implemented solutions. To this end, we extend a data-driven constraint customization framework in the literature which has been developed to extract constraints in the form of a traditional linear regression model to the case when constraints take the form of decision trees. This allows us to learn and incorporate customization constraints in a non-linear or logical form. To demonstrate the value of this data-driven constraint customization framework, experiments were conducted on the knapsack and nurse rostering problems where various combinations of hidden operational rules were simulated.
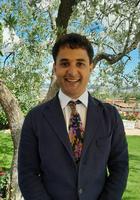
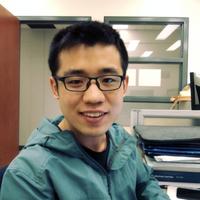
Location
Pavillon André-Aisenstadt
Campus de l'Université de Montréal
2920, chemin de la Tour
Montréal Québec H3T 1J4
Canada