Adaptive Partitioning for Chance-Constrained Problems
Marius Roland – Polytechnique Montréal, Canada
This presentation considers chance-constrained stochastic optimization problems (CCSPs) with finite support. We present an iterative algorithm that solves a reduced size chance-constrained model. This reduced model is obtained by partitioning the scenario set and, when solved to optimality, yields a bound on the optimal objective value of the original CCSP. Moreover, the algorithm ensures finite termination at an optimal solution of the original CCSP.
At the heart of the algorithm lie two fundamental operations: refinement and merging. Refinement involves splitting a subset of the partition, while merging combines two subsets. These operations are executed to enhance the bound obtained in each step of the algorithm while minimally increasing the size of the reduced model to be solved. Under mild conditions, we prove that, for specific refinement and merge operations, the bound obtained after solving each reduced model strictly improves throughout the iterative process. Furthermore, the algorithm structure allows for the seamless integration of various computational enhancements, significantly reducing the computational time required to solve the reduced chance-constrained problems. Based on theoretical arguments we also provide strategies to initialize the partition considered in the algorithm. Further, we make a parallel with the well studied quantile cuts, leading to a new family of stronger valid inequalities.
The efficiency of the algorithm is assessed through numerical experiments. We study the impact of every component of the algorithm and compare its performance against state-of-the-art methods on chance constrained multidimensional knapsack problems.
Biography: I studied Mathematical Engineering at the Université catholique de Louvain, Belgium, where I received my Bachelor’s degree in 6/2017 and my Master’s degree in 6/2019. Afterwards, I received a PhD from Universität Trier where I was supervised by Prof. Martin Schmidt. During my PhD studies my research focused on adaptive refinement algorithms for optimization problems arising in energy transport networks. Recently, I started a Postdoctoral position at Polytechnique Montreal supervised by Prof. Thibaut Vidal. I am interested in doing research at the interface of optimization, artificial intelligence, and energy networks. A specific topic for which I currently show a lot of interest is exact algorithms constructed to iteratively solve smaller size surrogate of models that approximate a more realistic but hard to solve model.
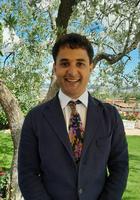
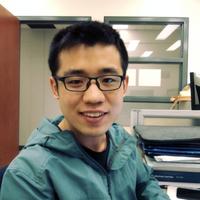
Location
Pavillon André-Aisenstadt
Campus de l'Université de Montréal
2920, chemin de la Tour
Montréal Québec H3T 1J4
Canada