High-dimensional black-box optimisation in small data regimes
Haitham Bou Ammar – Huawei Research London, United Kingdom
Many problems in science and engineering can be viewed as instances of black-box optimisation over high-dimensional (structured) input spaces. Applications are ubiquitous, including arithmetic expression formation from formal grammars and property-guided molecule generation, to name a few. Machine learning (ML) has shown promising results in many such problems (sometimes) leading to state-of-the-art results. Abide those successes, modern ML techniques are data-hungry, requiring hundreds of thousands if not millions of labelled data. Unfortunately, many real-world applications do not enjoy such a luxury -- it is challenging to acquire millions of wet-lab experiments when designing new molecules.
This talk will elaborate on novel techniques we developed for high-dimensional Bayesian optimisation (BO), capable of efficiently resolving such data bottlenecks. Our methods combine ideas from deep metric learning with BO to enable sample efficient low-dimensional surrogate optimisation. We provide theoretical guarantees demonstrating vanishing regrets with respect to the true high-dimensional optimisation problem. Furthermore, in a set of experiments, we confirm the effectiveness of our techniques in reducing sample sizes by acquiring state-of-the-art logP molecule values utilising only 1% labels compared to previous SOTA.
Bio: Haitham leads the reinforcement learning team at Huawei technologies Research & Development UK and is an Honorary Lecturer at UCL. Prior to Huawei, Haitham led the reinforcement learning and tuneable AI team at PROWLER.io, where he contributed to their technology in finance and logistics. Prior to joining PROWLER.io, Haitham was an Assistant Professor in the Computer Science Department at the American University of Beirut (AUB). Before joining the AUB, Haitham was a postdoctoral research associate in the Department of Operational Research and Financial Engineering (ORFE) at Princeton University. Prior to Princeton, he conducted researcher in lifelong machine learning while being employed as a postdoctoral researcher at the University of Pennsylvania. Being a former member of the General Robotics Automation Sensing and Perception (GRASP) lab, he also contributed to the application of machine learning to robotics. His primary research interests lie in the field of statistical machine learning and artificial intelligence, focusing on Bayesian optimisation, probabilistic modelling and reinforcement learning. He is also interested in learning using massive amounts of data over extended time horizons – a property common to "Big-Data" problems. His research also spans different areas of control theory and nonlinear dynamical systems, as well as social networks and distributed optimization.
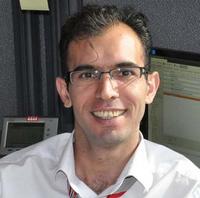
Location
Montréal Québec
Canada